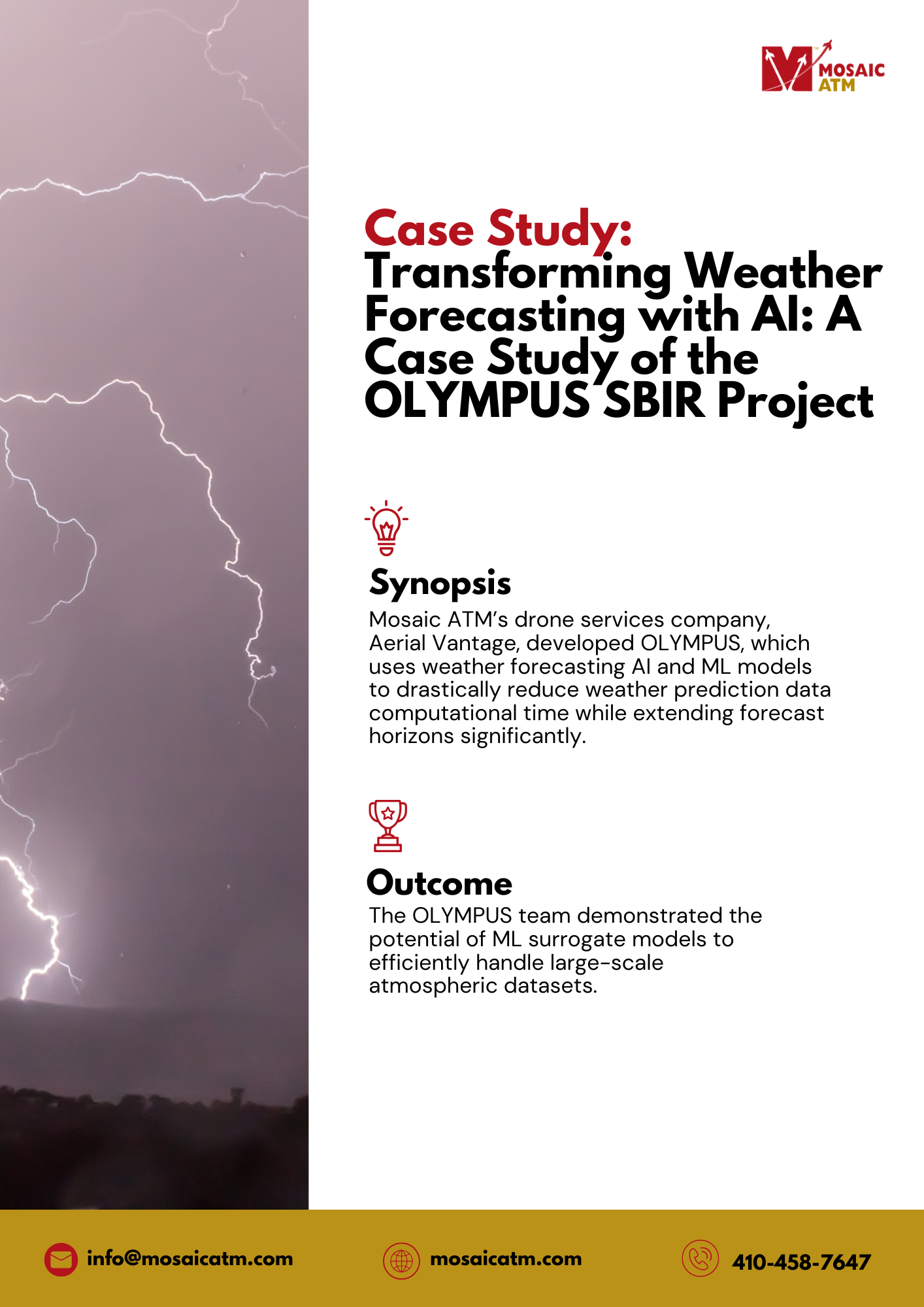
Take Our Content to Go
Mosaic ATM’s drone services company, Aerial Vantage, developed OLYMPUS, which uses weather forecasting AI and ML models to drastically reduce weather prediction data computational time while extending forecast horizons significantly.
Introduction
Weather prediction is crucial for a multitude of industries ranging from agriculture and aviation to energy and disaster management. Despite advances in forecasting, current physics-based Numerical Weather Forecasting (NWF) systems have two major drawbacks:
- They are computationally demanding, requiring hours of compute time on a high-performance-computing cluster.
- They are typically limited in accuracy to a 14-day forecast horizon due to the chaotic nature of weather.
Recognizing these challenges, Mosaic ATM initiated a NASA Small Business Innovation Research (SBIR) project under Aerial Vantage. The Optimized Learning for Yielding Meteorological Predictions Using Surrogates (OLYMPUS) initiative aims to revolutionize forecasting skill and computational efficiency through machine learning (ML) surrogate models.
The Problem: Beyond the Computational Limits
Traditional numerical weather forecasts, though reliable for short-term predictions, are computationally demanding and often struggle with error accumulation beyond 14 days due to their sequential prediction methodologies. A single time-step error can exponentially amplify, significantly affecting long-term forecast accuracy. Thus, industries reliant on precise long-range forecasts have been underserved by existing methodologies.
The Solution: AI-Driven Surrogate Models
Mosaic ATM’s drone services company, Aerial Vantage, developed the OLYMPUS project, which uses ML models to drastically reduce computational time while extending forecast horizons significantly. Many modern deep learning model architectures simply don’t scale to the size of a global Numerical Weather Prediction (NWP). Because of this, ML-based weather prediction (MLWP) systems either focus on localized regions or use custom models.
Our surrogate models leveraged advanced dimensionality reduction techniques, achieving an extraordinary 10,000:1 data compression ratio while preserving critical atmospheric information. This allows us to use Transformer-based architecture that can take advantage of many time steps.
Achievements, Validation and Insights
During Phase I, the OLYMPUS team demonstrated the potential of ML surrogate models to efficiently handle large-scale atmospheric datasets. Key achievements included:
- Successfully compressing data by a factor of 10,000 with minimal loss in accuracy, reducing the original data dimensions dramatically.
- Training advanced Transformer-based models on 9 years of historical GEOS-FP data, cutting validation losses significantly and enhancing long-term predictive stability.
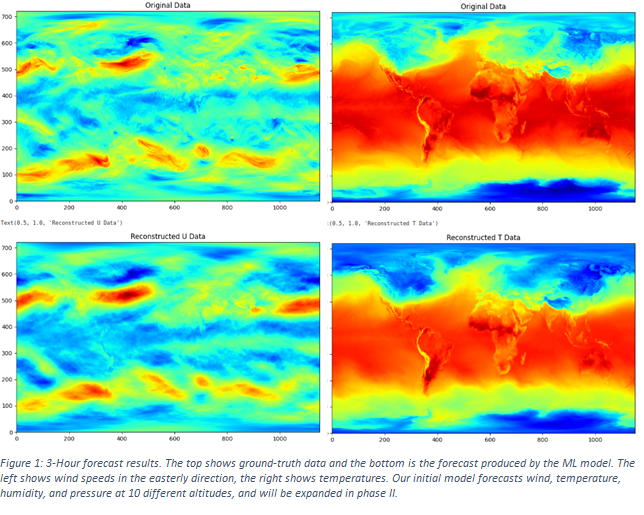
OLYMPUS’s predictive performance showed promise in initial evaluations:
- The ML surrogate models demonstrated rapid forecasting capabilities, offering results in minutes versus hours or days required by traditional physics-based systems.
- Forecast accuracy improved substantially with increased data availability, showing an error reduction of over 50% when the training dataset expanded from two to nine years.
Commercialization: Bridging Science and Industry
OLYMPUS leveraged customer discovery insights from agricultural cooperatives, aviation experts, and energy providers to tailor its forecasting platform to specific industry needs. Farmers particularly benefit from precise weather forecasts for critical activities like planting, spraying, and harvesting—tasks highly sensitive to accurate weather predictions.
The commercial strategy includes:
- Pilot testing with agricultural end-users to refine the forecasting model for operational reliability.
- Subscription-based access to long-range forecasts delivered through a user-friendly web application, allowing easy integration into existing decision-making processes.
- Strategic partnerships and licensing models aimed at expanding market reach and creating scalable revenue streams.
Looking Ahead: The Next Generation of Forecasting
In its next phase, OLYMPUS will wield the power of weather forecasting AI to:
- Further enhance model accuracy and forecasting horizons, targeting reliable predictions up to 28 days ahead.
- Implement multi-GPU distributed training techniques to manage larger models and more complex atmospheric datasets efficiently.
- Integrate uncertainty quantification tools to improve forecast reliability and actionable insights.
- Pilot the technology extensively with targeted industry stakeholders to refine usability and demonstrate its practical benefits in operational environments.
The OLYMPUS project represents not just an incremental improvement but a transformative shift in how weather predictions are generated and utilized, aligning closely with NASA’s goals in Earth science, atmospheric modeling, and the practical application of artificial intelligence.